How It's Made
Your Fully Personalized Grocery Store: How Ads can improve your online shopping experience
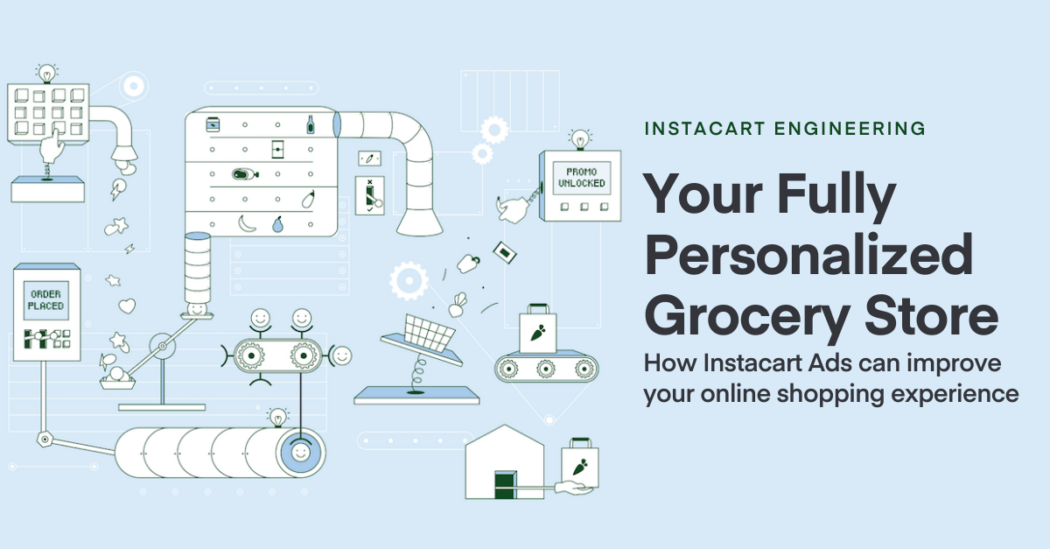
Written by Girija Narlikar, Engineering Director, Ads Quality
Imagine your favorite neighborhood grocery store, except that it’s arranged especially for you on every visit. Instacart’s ads platform is powering a marketplace filled with digital stores that can help you locate your favorite products with ease, discover new items to your taste, and inspire you with rich food related content–all without leaving the comfort of your home.
We’ve established our core product search capability, matched with relevant Sponsored Products. We’ve now launched a self-service display ads offering that mimics supermarket checkout shelves and endcap displays. These ads are intended to inspire customers with a highly personalized product discovery experience along their shopping journey.
Traditionally, the consumer packaged goods (CPG) industry has relied on media like TV, radio, and magazines (both print and online). However, these forms of media have limited personalization and real-time measurement capabilities. As a platform with a complete view of a customer’s food awareness and purchase journey, Instacart is in a unique position to learn from, and predict, customer preferences in a privacy-safe manner. This also allows our CPG advertisers to measure the end-to-end impact of their sponsored campaigns. As more consumers adopt online grocery shopping, Instacart is an increasingly important destination for CPGs to connect with their target audience in ways personalized to each individual customer.
How do we provide this personalized service to both customers and advertisers? Let’s outline how the Display Ads team learns customer behavior and tailors content delivery methods, while identifying some interesting challenges to tackle in the coming year.
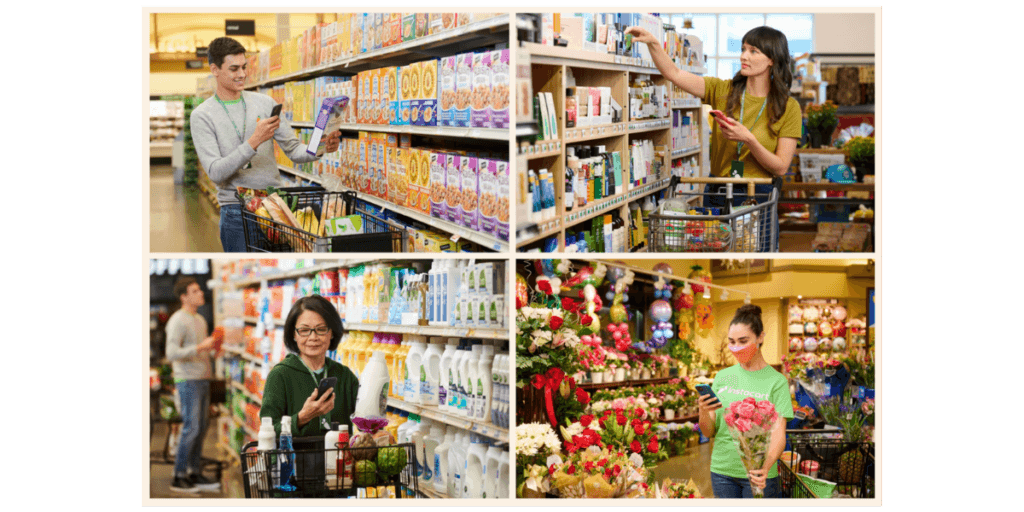
Understanding our customers and advertisers to create audiences
Instacart learns over time what products each customer is likely to be interested in. Based on past purchase patterns, including individual ingredients and finished food products, we can learn about the customer’s or household’s food preferences. The Ads team is building ML-based capabilities to connect advertisers to those sets of customers that are most likely to find the advertiser-sponsored content engaging and useful, maximizing both advertiser and customer value.
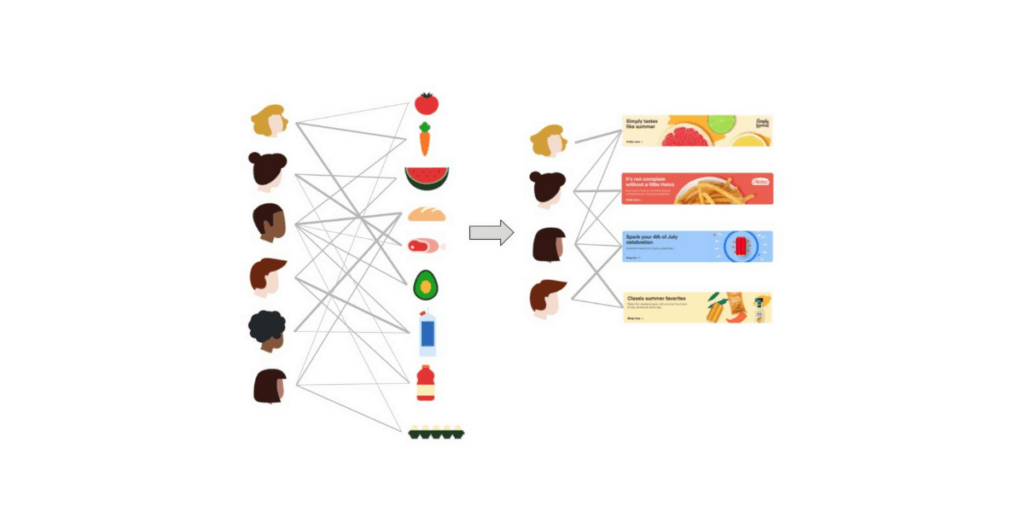
One of the signals used to build audiences is the Instacart personalization model. The model is a two-tower DNN [1][2] which generates customer and product embeddings in the penultimate layer. A dot product between the embeddings from the customer and product towers is used to predict if a customer has an interest in a product from a retailer. The model is trained on positive examples generated from actual customer purchases on Instacart and compared against products in the product catalog that were not purchased. It takes historical customer purchases, dietary preferences, and other customer features as inputs to the customer tower while product metadata and popularity serve as inputs to the product tower. The customer and product embeddings are recomputed periodically to reflect changing customer preferences and capture product purchase trends. We’re currently using this model to optimize a customer’s browsing experience on Instacart and as input into other ML models used to power search, ads, and more.
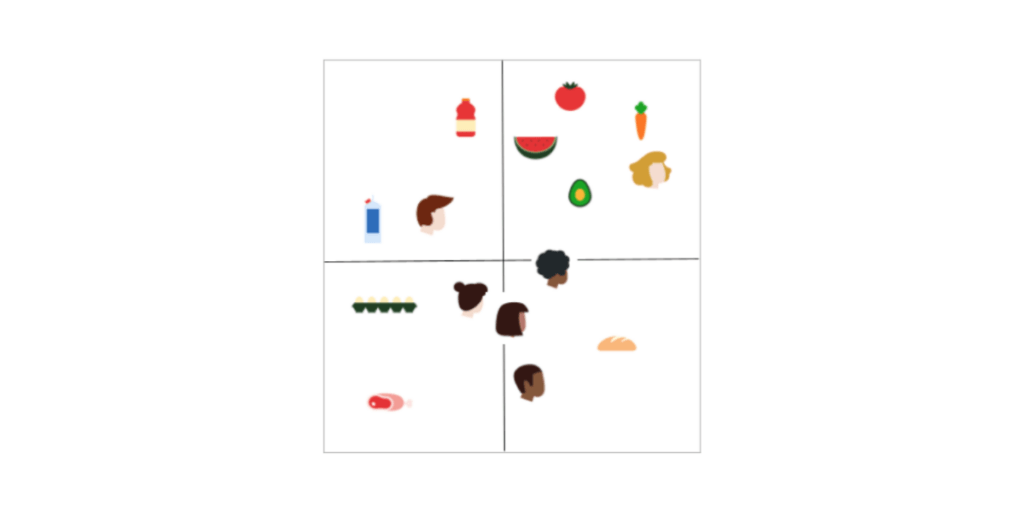
Personalization of sponsored product recommendations on Instacart poses multiple challenges. Each Instacart customer may either represent themselves or an entire household. They may also have strong, varied product preferences depending on the retailer. Some foods may be popular predominantly as seasonal or festive items. Some customers may be heavily influenced by savings offers. New customers have little history on Instacart, presenting challenging cold start problems. We also find that customers on Instacart go through phases of strong intent (in search of specific products or product categories), balanced with exploration and discovery when they are open to broader suggestions. This means that we need to balance strong personalization and intent signals with broader exploration, novelty, and diversity of product recommendations.
Meanwhile, advertisers typically have distinct goals for different campaigns, each with its own creative content, messaging, keywords, and audience selection. For example, some campaigns may aim to retain loyal customers, while others may strive to bring back lapsed customers or attract new customers. By understanding the various advertising goals for each campaign as well as customers’ preferences, Instacart Ads can automatically optimize and adjust the audiences that are preferentially shown ads from each campaign.
Recommendations for food related content
While Instacart provides a very efficient surface to search for and purchase items, it can also support a native, content-heavy experience with interactive images, videos, recipes, and food blogs. Just as people look forward to discovering new products or being inspired by new ideas when they visit physical stores, rich content on the Instacart App can help CPGs effectively connect with and delight customers in a personalized and timely manner.
Understanding and predicting how customers react and interact with such content will be a large part of our efforts in 2022. For example, we may discover some customers are interested in baking or barbeque, while others prefer vegan recipes. Easy-to-assemble meal kits can add more value to busy parents of school-aged children, while baby food tips and diaper coupon offers will be useful to new parents. Some users may be more intrigued than others to discover new brands through videos. Instacart can learn these preferences over time, but it can also “warm start” content recommendations based on past behavior and inherent properties of the content itself.
Recipes themselves present a trove of technical challenges–extracting ingredients as structured data, identifying key ingredients, finding similar recipes, suggesting substitutions for dietary restrictions, and recommending the right recipes, keeping in mind factors such as dietary preferences and seasonal popularity. Offline, CPGs have long used “back-of-the-box” recipes or tasting booths to highlight the usefulness and convenience of their in-store products. Online, we can provide these same CPGs with a way to showcase products to customers in the comfort of their homes.
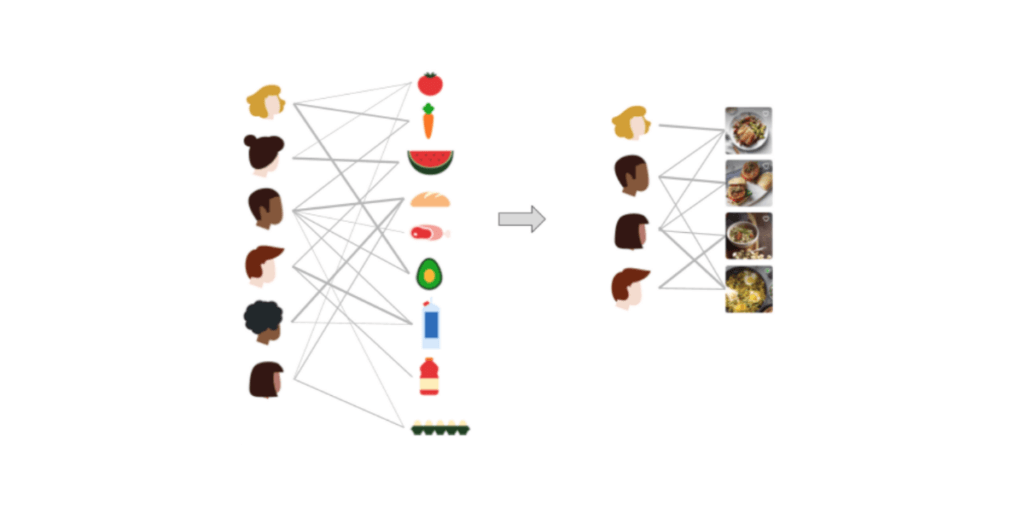
Personalized content assembly
As Instacart Ads advances its products in the rich content space, optimizing the right combination of sponsored content across the page for each customer will become a critical task. This will entail selecting the media type, assets, and audience targeting levers to create relevant and inspiring experiences based on long-term and recent history, current serving context, and content features.
With infinite possibilities of customer needs and content delivery methods, Instacart display ads enables CPGs to reach customers in a natural, integrated way. The Instacart Ads team has identified the priorities and challenges in delivering the best personalized grocery store that naturally blends advertising messaging into the customer experience.
References
[1] Paul Covington, Jay Adams, Emre Sargin, “Deep Neural Networks for YouTube Recommendations”, RecSys ’16 September 15-19, 2016, Boston , MA, USA
[2] Malay Haldar, Prashant Ramanathan, Tyler Sax, Mustafa Abdool, Lanbo Zhang, Aamir Mansawala, Shulin Yang, Bradley Turnbull, Junshuo Liao, “Improving Deep Learning For Airbnb Search”.
Most Recent in How It's Made

How It's Made
One Model to Serve Them All: How Instacart deployed a single Deep Learning pCTR model for multiple surfaces with improved operations and performance along the way
Authors: Cheng Jia, Peng Qi, Joseph Haraldson, Adway Dhillon, Qiao Jiang, Sharath Rao Introduction Instacart Ads and Ranking Models At Instacart Ads, our focus lies in delivering the utmost relevance in advertisements to our customers, facilitating novel product discovery and enhancing…...
Dec 19, 2023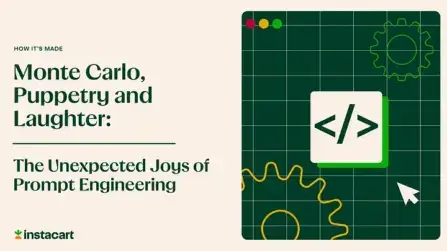
How It's Made
Monte Carlo, Puppetry and Laughter: The Unexpected Joys of Prompt Engineering
Author: Ben Bader The universe of the current Large Language Models (LLMs) engineering is electrifying, to say the least. The industry has been on fire with change since the launch of ChatGPT in November of…...
Dec 19, 2023
How It's Made
Unveiling the Core of Instacart’s Griffin 2.0: A Deep Dive into the Machine Learning Training Platform
Authors: Han Li, Sahil Khanna, Jocelyn De La Rosa, Moping Dou, Sharad Gupta, Chenyang Yu and Rajpal Paryani Background About a year ago, we introduced the first version of Griffin, Instacart’s first ML Platform, detailing its development and support for end-to-end ML in…...
Nov 22, 2023